图技术全景
Graph technology is built to handle complex networks of data, surfacing connections and highlighting patterns and anomalies far more efficiently than more traditional technologies. In a world where organizations rely on increasing amounts of data for critical business decisions, high-stakes investigations, and more, it’s no wonder that graph has been gaining more traction.
Graph technology is still a relatively young domain - which means it’s still evolving a lot. Our current context of big data and increasingly data-driven business operations has in turn driven growth and a lot of innovation in the sector, as a wide variety of organizations have come to see the value that graph technology can provide in delivering deeper insights that were previously inaccessible.
Graph technology: quickly evolving and expanding
We originally published an introduction to the graph technology landscape back in 2014. Linkurious was still young. And Google’s PageRank algorithm and Facebook’s Graph Search were still fresh examples of what you could do with graph technology. At the time we described the graph ecosystem as “emerging”, and there were far fewer tools available on the market.
The graph technology market has vastly expanded since then as existing companies have matured and built on their graph products, and as new players have emerged. Graph enthusiasts and graph companies have also made major strides in further democratizing graph technology.
The result has been that more and more organizations are adopting graph technology, as they find it to be an asset for an ever increasing number of use cases. We’ll be honest, this has been a thrill to watch over the years as the graph industry finds new ways to deliver value for all kinds of applications. We now see graphs being used for cybersecurity, drug discovery, finance, anti-money laundering, intelligence, manufacturing, IT management… the list goes on.
We’re not the only ones to notice this, of course. The proof is in the market growth. The graph database market is expected to grow to a value of US$ 3.78 billion by 2027, up from US$ 1.13 billion in 2021.
Gartner has also observed the ways in which graph serves modern enterprises and has predicted that this technology will continue to grow. “Graph forms the foundation of modern data and analytics with capabilities to enhance and improve user collaboration, machine learning models and explainable AI,” Gartner writes in their Top 10 Data and Analytics Trends for 2021.“
Graph and AI
Another factor driving the popularity of graph technology is its ability to enhance various artificial intelligence (AI) applications. This includes generative AI applications like large language models (LLMs), whose accuracy can be improved using knowledge graphs. “Although graph technologies are not new to data and analytics, there has been a shift in the thinking around them as organizations identify an increasing number of use cases,” writes Gartner. “In fact, as many as 50% of Gartner client inquiries around the topic of AI involve a discussion around the use of graph technology.”
Understanding the graph technology landscape
With all this change and increasing growth, it seemed high time to revisit the graph technology overviews we published in 2014 and in 2019. Our goal is to introduce you to the key categories within the world of graph tech, and to the key players within those categories, as we’ve seen them emerge and evolve over our 10+ years of experience in the field. We cover tools all across the graph data value chain, from data injection to end-user analytics. To help you understand where each of these tools fits along the value chain, we’ve drawn up a diagram to keep in mind as you read.
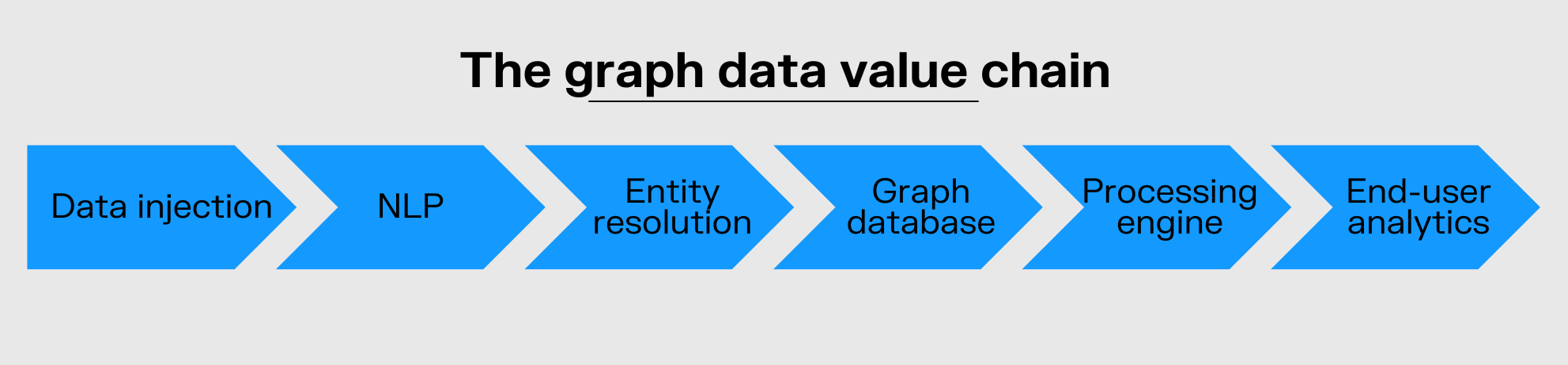
To be clear, this list is not all encompassing. The graph technology landscape has become very large and is evolving quickly, so it would be tough to capture a complete picture - and even if we did, it would soon be outdated by the many changes that are happening all the time in this field. Instead, we’re delivering an overview of the main tools and solutions on the market, alongside some important trends to keep an eye on. To keep things simple and (relatively) concise, we’ve limited the list to companies of at least 15 people.
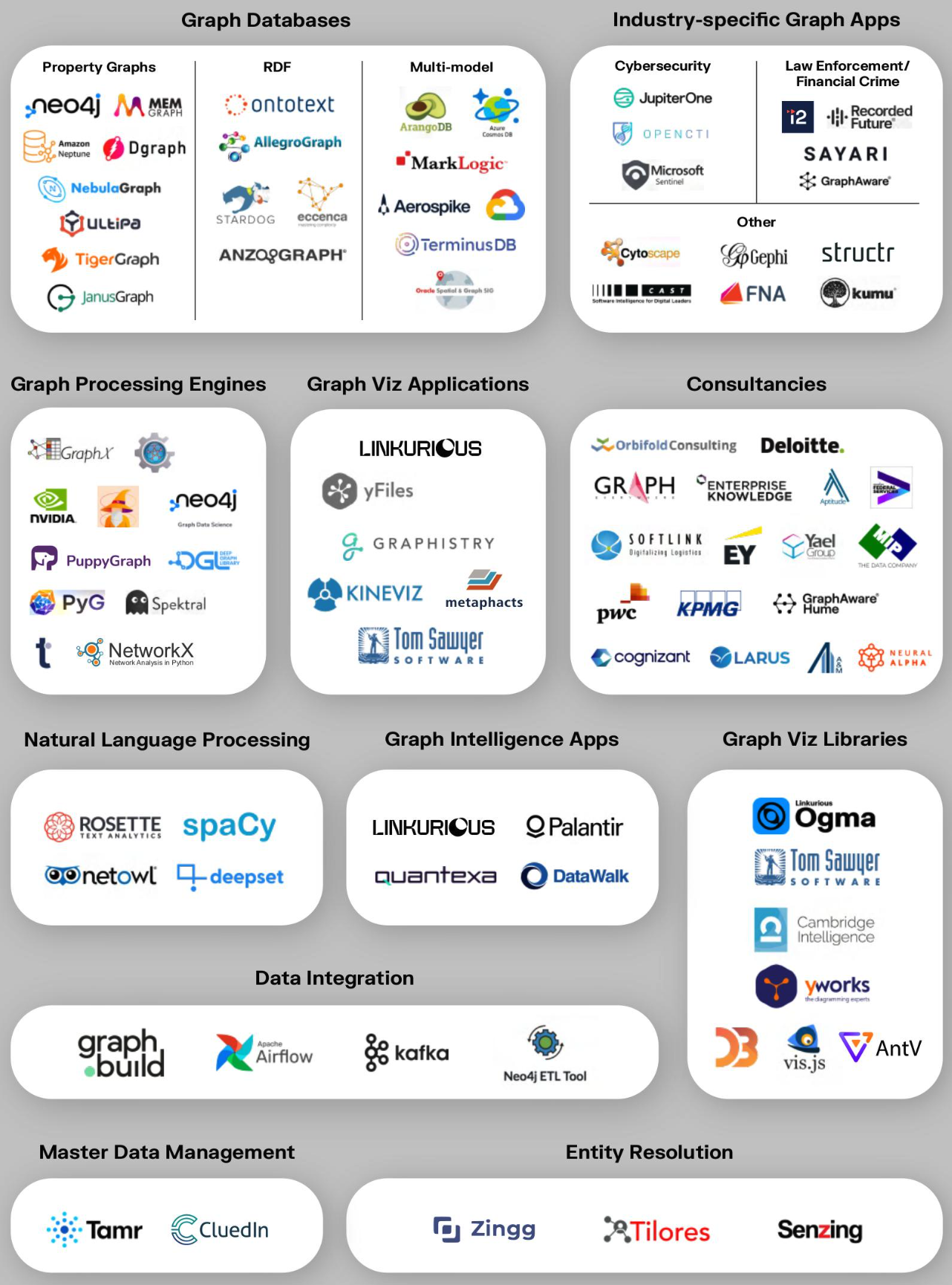
The graph technology landscape in 2025
Graph databases
When discussing the graph technology landscape, graph databases are the logical place to start. Graph databases are foundational for the adoption of graph technology. These systems help organizations tackle the technical challenges of storing complex connected data and extracting insights from very large datasets. If you have a graph application, chances are that you will require a graph DB.
There are several types of databases that are designed for graph data, which include property graph databases, RDF databases, multi-model databases, and high-performance computing databases. We’ll briefly get into what defines each of these categories below. There are fewer new players today than there were some years back, but the graph database space remains active. Google is the most recent big player to enter the ring, announcing their Spanner Graph in August 2024.
There is an increasing offer of cloud graph databases, many of them coming from major providers already established in the graph space, supporting teams building cloud-based applications.
Despite growth in the sector, the graph DB space isn’t without its challenges. Certain graph database companies have recently seen layoffs. Redis announced the end of life of RedisGraph in mid-2023.
And as with any newer technology sector, the space is still shifting. Some of the most recent changes are related to query languages. Graph Query Language Standard (GQL), a standardized query language for property graphs, was released in April 2024. And Property Graph Query Language (PGQL) is a standard built on top of SQL, that brings graph queries to SQL databases.
Finally, we are seeing more and more SaaS offerings among graph databases.
Property graphs
Property graphs are entirely optimized to work with graph-like data. Here, Neo4j is the market leader, having launched their first native graph database in 2010. Since then several other players have emerged on the market.
- Neo4j. Their property graph database remains the most popular one. A closed source database, Neo4j exists in both on-premises and cloud versions (Neo4j Aura). Neo4j uses Cypher query language.
- Amazon Neptune. Amazon Neptune is closed source and is available both on-premises and on the cloud. Neptune supports graph models including Property Graph and W3C’s RDF and their query languages: Apache TinkerPop Gremlin, SPARQL and OpenCypher.
- Memgraph. Memgraph, publicly available since 2017, is a graph streaming platform built on top of an in-memory graph database. It is open source and uses Cypher query language. Memgraph can be run locally, on-prem or as a managed service through Memgraph Cloud.
- TigerGraph. This is a hybrid transactional/analytical processing database and analytics software, implemented in C++. It has its own graph query language similar to DQL called GSQL.
- JanusGraph. Originally called “TitanDB”, JanusGraph is the open-source project that invented the Gremlin query language. They provide a compatibility layer that allows to turn almost any Key-Value store into a distributed graph database.
- NebulaGraph. This is an open source, distributed, and easily scalable graph database that uses nGQL query language, and more recently GQL. It’s built for super large-scale graphs and can process trillions of nodes and edges.
- DGraph. DGraph is an open source native and distributed graph database, with native GraphQL support. It uses DQL, DGraph’s proprietary query language.
- Ultipa. This graph database and knowledge graph system supports real-time computing and analytics. Ultipa is closed source and uses a proprietary query language called UQL.
RDF
An RDF triplestore is a category of graph database in which data is stored as a network of objects. They use inference to discover new information from existing relations.
- OntoText (Graphwise). This is a graph database and knowledge discovery tool compliant with RDF and SPARQL. It recently merged with Semantic Web Company (SWC) to create a graph platform that combines knowledge graphs and semantic AI capabilities.
- Stardog. Stardog is a knowledge graph platform and graph DBMS with high availability and performance. It combines graph database technology with an AI-based knowledge toolkit.
- AllegroGraph. A high-performance, persistent RDF store with support for graph DBMS. AllegroGraph supports queries through SPARQL, Prolog and languages like JavaScript.
- AnzoGraph. This is a scalable graph database built for online analytics and data harmonization with MPP scaling, high-performance analytical algorithms and reasoning, and virtualization.
- Eccenca. An enterprise knowledge graph platform that helps scale use of knowledge in automatic decisions across processes.
Multi-model
Multi-model databases emerged as an answer to the complexity that the multiplication of siloed systems was creating. These databases are designed to support various data types, handling in one single data store various models such as document, key-value, RDF and graphs. They are particularly convenient if you need to work with multiple data types but want to avoid the operational complexity of managing various silos.
- ArangoDB. This is a native multi-model, open-source database with flexible data models for documents, graphs and key-values.
- Microsoft Azure Cosmos DB. This is a fully managed NoSQL database for modern app development, available on-prem and on the cloud. Users can choose between document mode or graph mode, and in the latter, you can query your graph in SQL or Gremlin.
- Oracle Spatial and Graph. This product includes a property graph database with built-in graph analytics, and a range of spatial analysis functions. It uses PGQL query language and newly supports GQL.
- Google Spanner Graph. Spanner Graph supports a graph query interface compatible with GQL. It supports interoperability between relational and graph models, combining SQL capabilities with graph pattern matching from GQL.
- MarkLogic. MarkLogic is a historical stakeholder with a document-oriented database. It evolved from an XML database to natively store JSON documents and RDF triples.
- TerminusDB. This is an open source knowledge graph and document store, used to build versioned data products.
- Aerospike. The Aerospike database is a real-time data platform for multi-cloud JSON and SQL use cases.
Graph processing engines
Graph processing engines are the systems used to compute graph data. Some graph processing engines and graph databases exist in closely connected ecosystems. Neo4j GDS, Neptune ML, and Memgraph MAGE are all deeply integrated with the Neo4j graph database, Amazon Neptune and the Memgraph graph database respectively. In this category there are also tools that work with non-graph database backends, such as Apache Spark and NetworkX. Some libraries are focused on neural networks specifically. These include DGL, Spektral, and PyTorch Geometric.
- GraphX. Introduced in 2014, GraphX is the embedded graph processing framework built on top of Apache Spark for parallel computing.
- Microsoft GraphEngine. A distributed in-memory data processing engine, underpinned by a strongly-typed RAM store and a general distributed computation engine.
- Neo4j GDS. An analytics and machine learning solution that analyzes the relationships within data.
- Neptune ML. This works with Amazon Neptune and uses Graph Neural Networks to make easy, fast, and more accurate predictions using graph data.
- Memgraph MAGE. An open-source repository that contains graph algorithms and modules in the form of query modules that extend the OpenCypher query language.
- PuppyGraph. A scalable graph query engine that allows users to query one or more of their existing relational data stores as a unified graph via Cypher and Gremlin.
- Graphstorm. Launched by AWS in 2023, GraphStorm is a low-code graph machine learning framework to build, train, and deploy graph ML solutions.
- NVIDIA cuGraph. A collection of GPU accelerated graph algorithms that process data found in GPU DataFrames. cuGraph supports cuDF DataFrame and Pandas DataFrame for graph creation.
- NetworkX. A Python package for the creation, manipulation and study of the structure, dynamics and functions of complex networks.
- Deep Graph Library. DGL is a Python package that provides easy implementations of graph neural networks (GNN) research. DGL integrates with existing major deep learning libraries including PyTorch and MXN.
- Spektral. A Python library for graph deep learning, based on the Keras API and TensorFlow 2. It provides a simple but flexible framework for creating graph neural networks.
- PyG (Pytorch Geometric). A library built with PyTorch to write and train graph neural networks for a range of applications.
- ThatDot. thatDot is a scalable streaming graph event processing engine designed for real-time, massively parallel, graph data processing and analytics.
Creating the graph
As the graph technology space is maturing, there are more and more tooling options available within the ecosystem. An increasing number of tools are now dedicated to the integration of graph data. To give an example, there are now connections to standard graph databases in popular ETL tools. Kafka supports Neo4j and TigerGraph for instance.
In this category, we have also regrouped tools that are adjacent to graph technology. They can be used to process graph data or other data structures. There is natural language processing (NLP) for instance, which is necessary as soon as you start working with unstructured data. Or entity resolution, which is a necessity when you’re working with multiple data sources and need to ensure your data quality.
Data integration
- Graph.Build. A collaborative, no-code platform used to design, configure, and automate graph model production.
- Apache Hop. An open source, metadata-driven data engineering and data orchestration platform that lets you visually describe your data pipelines and workflows.
- Apache Airflow. An open-source workflow management platform for data engineering pipelines. Workflows are created via Python scripts.
- Kafka. The Kafka Connect Neo4j Connector is an extension developed to integrate Kafka and other streaming solutions with Neo4j.
- Neo4j ETL Tool. Extracts the schema from any relational database to turn it into a graph schema, and imports data into a graph in either bulk or online mode.
Natural language processing (NLP)
- NetOwl. An AI-enabled multilingual text analysis solution used to analyze unstructured big data as well as structured entity data.
- Rosette. Rosette processes and analyzes large amounts of text across multiple languages and performs name matching using AI.
- Deepset. Deepset provides developers with tools to build natural language processing systems.
- spaCy. An open source software library for advanced natural language processing written in Python and Cython.
Entity resolution (ER)
- Senzing. An API for developers integrating ML that makes it easy to integrate entity resolution into workflows and architectures.
- Tilores. A data orchestration platform with no-code identity resolution technology for fraud prevention, KYC and AML teams.
- Zingg. An ML-based tool for entity resolution to create a single source of truth for business entities.
Master data management (MDM)
- CluedIn. A graph-based, Azure-native MDM platform that lets users prepare, govern, and share both structured and unstructured data.
- Tamr. Tamr enables users to consolidate messy source data into clean, curated, analytics-ready datasets.
Graph applications
When we first published our introduction to the graph technology landscape, Linkurious was already a pioneer, standing alone in offering a business-friendly graph visualization and analytics tool. Now, there are more tools on the market; there has been a lot of innovation around general purpose graph visualization tools over the last few years.
As a pioneer with a long experience however, Linkurious remains the preferred off the shelf visualization tool for hundreds of clients and thousands of users. It benefits not only technical users but also non technical users because of its intuitive user interface, interoperability with leading graph databases, and versatility.
Graph intelligence solutions
The last several years have also seen the emergence of graph intelligence solutions like Palantir or Quantexa, now joined by the Linkurious Decision Intelligence Platform. Going beyond simple graph visualization, these tools use advanced algorithms, machine learning, entity resolution, and data analysis techniques to provide actionable intelligence, identifying patterns, anomalies and trends within interconnected data to detect and investigate fraud, money laundering, intelligence threats, etc.
Industry-specific tools
There are also a number of industry-specific tools now available on the market. This category can be difficult to delimit as some products integrate graph technology in different ways. There are products that use a graph database in the backend, and where the primary user interface is a graph visualization UI. Cartography is an example of this.
On the other hand, there are tools that take a more hybrid approach. Maybe the backend doesn’t use a graph database, or maybe a graph UI is just one way to access the data. This in itself is interesting: it’s a concrete illustration of how pervasive the graph approach has become.
Graph visualization applications
- Linkurious. A graph visualization and analytics tool with an intuitive UI, compatible with all leading graph DBs, that’s accessible to both technical and non-technical users, enabling them to quickly and easily explore, analyze, and visualize their complex, connected graph data. Including advanced filtering, timeline and geo-layout features, robust teamwork features, and more, Linkurious is the #1 graph visualization tool being used by Fortune 500 companies, with thousands of users.
- Neo4j Bloom. A graph visualization and analytics tool that functions within the Neo4j ecosystem and works well for small teams who want to visually explore their data.
- yFiles Neo4j Explorer. A free browser tool that lets you interactively explore your Neo4j database.
- Kineviz GraphXR. A browser-based visualization platform for interactive analysis of big, high-dimensional, or connected data.
- Graphistry. Built with end-to-end GPU and visual graph analytics, Graphistry lets users transform graph data into interactive graph visualizations.
- Metaphacts. A flexible, open platform for the creation, visualization, and exploration of knowledge graphs.
- Tom Sawyer Explorations. A graph intelligence application that lets users uncover insights through data integration, graph pattern matching, and graph visualization and analysis.
Graph intelligence applications
- Linkurious Decision Intelligence Platform. A fully modular decision intelligence platform powered by native graph technology, entity resolution, and AI that helps both technical and non-technical users understand the relationships and context within connected data. Linkurious includes powerful scoring and alerting features for more effective, accelerated decision making.
- Palantir. A data analytics tool that lets users integrate and analyze all their data for use cases ranging from national security and intelligence to anti-fraud to healthcare and more.
- Quantexa. A decision intelligence platform to connect siloed systems and visualize connected data. Quantexa uses networks and contextual monitoring to manage financial crime and fraud risk and detect, prevent, and investigate suspicious activity.
- DataWalk. Analysis software that brings together data sources to enable users to find patterns and connections for intelligence analysis, fraud detection, and more.
Industry-specific graph apps
Cybersecurity
- JupiterOne. A cyber asset analysis platform for cybersecurity to continuously collect, connect, and analyze asset data to make informed decisions about risk and security.
- OpenCTI. An open source platform for organizations to manage their cyber threat intelligence knowledge. Includes visualization features and case management.
- Cartography. A Python tool that consolidates infrastructure assets and the relationships between them in a graph view powered by Neo4j graph database.
- Microsoft Sentinel. A cloud-native security information and event management platform that uses AI to help analyze large volumes of data for threat detection, investigation, and response.
Law enforcement/financial crime
- i2. A platform that provides visual analysis capabilities that turn data into actionable intelligence to identify, predict, and prevent criminal, terrorist, and fraudulent activities.
- Sayari. Sayari Graph harvests public records from 200+ jurisdictions to deliver a global database of ownership hierarchies, commercial relationships, and risk analyses to mitigate risk exposure and fight financial crime.
- Recorded Future. A cybersecurity and intelligence solution that uses machine learning and natural language processing to collect and organize data from open web, dark web, and technical sources.
- GraphAware Hume. A government-grade data analytics solution for intelligence analysis with natural language processing and other machine learning capabilities.
Other
- Cast Software. Provides products that generate software intelligence, with a technology based on semantic analysis of software source code and components. Their technology automatically "understands" custom-built software systems and provides insights into their inner workings.
- Kumu. Kumu lets you organize complex data into relationship maps to capture different perspectives.
- structr. An integrated low-code development and runtime environment for web-based enterprise applications that leverages graph technology.
- FNA. Software that’s used to uncover hidden connections and anomalies in large, complex datasets to predict the impact of stress events for financial systems.
- Gephi. Gephi is a free, open-source network analysis and visualization software package that runs on Windows, Mac OS X, and Linux.
- Cytoscape. Initially designed as a platform for visualizing molecular interaction networks and biological pathways, Cytoscape is now a general, open-source platform for complex network analysis and visualization.
Visualization libraries
Graph visualization libraries are low-level building blocks that are used to build custom graph UIs. There are several well-established, open source libraries, including D3 and Vis.js. These libraries tend to lag in terms of performance and customization compared to the commercial offerings that are available on the market now.
Developers can also turn to a handful of strong commercial graph visualization libraries, including Linkurious’s own Ogma library, Keylines and Tom Sawyer.
Graph visualization libraries
- Ogma. This JavaScript graph visualization library leverages WebGL rendering and is a powerful solution to develop large-scale interactive graph visualizations. It features many layout algorithms, geo-mode, annotation layers, visual groupings, numerous styling options, and comes with many examples and templates. Ogma supports multiple integration frameworks for maximum flexibility.
- Keylines. This graph visualization engine supports canvas and WebGL rendering. It supports many graph algorithms and offers many different layouts. Keylines supports several integration frameworks and offers a specific one called ReGraph to speed up integration with React.
- Tom Sawyer Perspectives. A low-code graph visualization and analysis development platform. Integrated design and preview interfaces and extensive API libraries allow developers to create custom applications.
- YFiles. Developed by yWorks, this library enables users to visualize any kind of graph, diagram, or network. It supports a wide range of desktop and web technologies, as well as many layout algorithms.
- D3. This library is used to manipulate documents based on data using HTML, SVG, and CSS. There is no set integration framework so you have to build one yourself and render all behaviors, requiring a large time investment.
- Vis.js. This browser-based library is under the MIT license. It works with large, dynamic datasets. Vis.js offers common customization options for styling nodes, labels, animations, and more. The layouts and algorithms are limited, however.
- G6. This is a canvas-based graph visualization framework, developed by AntV, that integrates with React via its sister library Graphin. It’s well adapted for visualizing small to moderate sized graphs. G6 provides many algorithms and styling options. The implementations and API can be tricky, however, and parts of the documentation are in Chinese.